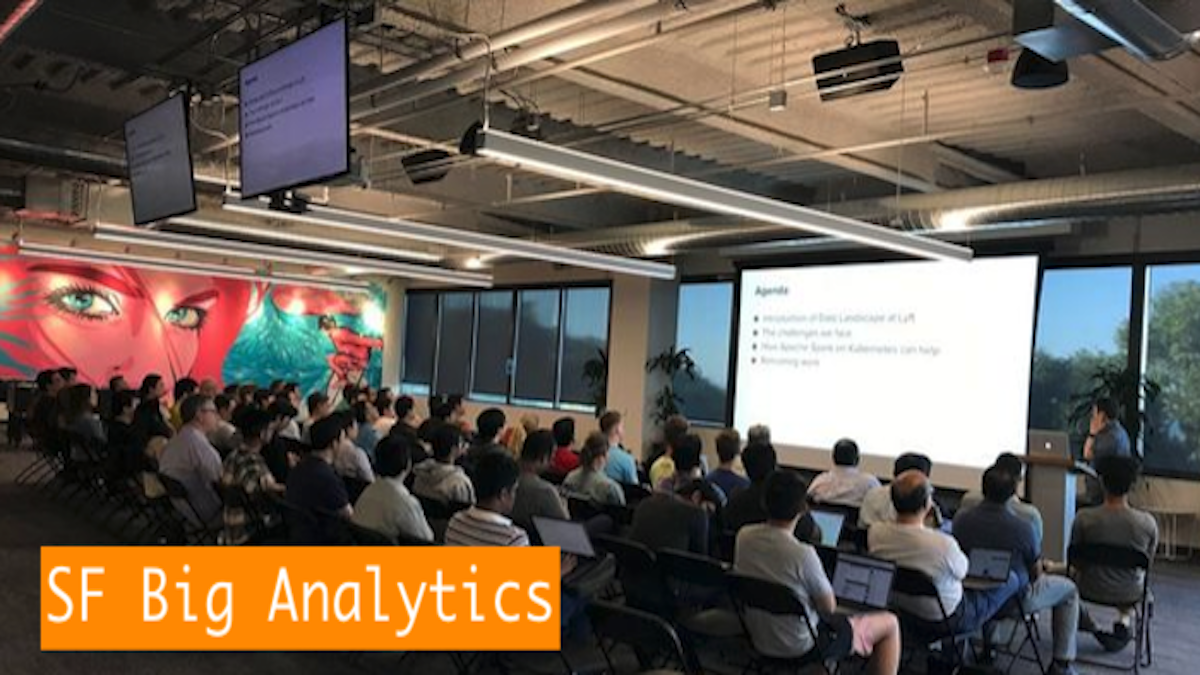
This virtual AI seminar is hosted by SF Big Analytics.
Tech Talk: Federated Learning with Public and Private Data: From Small Models to Large, and Back
Speaker: Zheng Xu (Google)
Abstract: In this talk, we will discuss federated learning in the cross-device and limited client resources settings. We consider the implication of model sizes and the usage of public and private data for practical applications. For on-device models in production, we train language models (LMs) with federated learning (FL) and differential privacy (DP) in the Google Keyboard (Gboard). We apply the DP-Follow-the-Regularized-Leader (DPFTRL) algorithm to achieve meaningfully formal DP guarantees without requiring uniform sampling of client devices.
Tech Talk: Federated Learning: Towards Real-world Studies
Speaker: Ziyue Xu (Nvidia)
Abstract: This talk will cover an end-to-end discussion for applying federated learning (FL) in real world studies – from theoretical algorithm design to practical framework implementation. Given the fundamentals of FL addressing the pivotal balance between data privacy and the collaborative enhancement of machine learning (ML) models, we will discuss the special challenges and solutions for embedding FL in AI model development. Specifically, we will talk about practical frameworks regarding system design and implementations, and further discuss the systematic requirements and features, especially in the age of LLMs. Ultimately, this talk underscores the transformative potential of FL in a general setting beyond deep learning, offering insights into its current achievements and future possibilities.
Tech Talk: pfl-research simulation framework for accelerating research in Private Federated Learning (PFL)
Speaker: Mona Chitnis (Apple)
Abstract: Federated Learning (FL) is an emerging Machine Learning (ML) paradigm where training data stays private on user/edge devices and aggregated gradients help us learn from a population. Differential Privacy (DP) is considered the industry standard for a rigorous privacy guarantee over statistics and model gradients. Large scale deployments of FL and DP are challenging but growing due to increased privacy awareness and regulations. On behalf of Apple, we introduce pfl-research, an open-source framework for PFL simulations, with a goal to equip and accelerate the research community, and improve reproducibility of FL dataset and algorithm benchmarks.
Sponsors:
We are actively seeking sponsors to support AI developers community. Whether it is by offering venue spaces, providing food, or cash sponsorship. Sponsors will not only speak at the meetups, receive prominent recognition, but also gain exposure to our extensive membership base of 350K+ worldwide.
Community on Slack/Discord
- Event chat: chat and connect with speakers and attendees
- Sharing blogs, events, job openings, projects collaborations
Join Slack (search and join the #virtualevents channel) | Join Discord