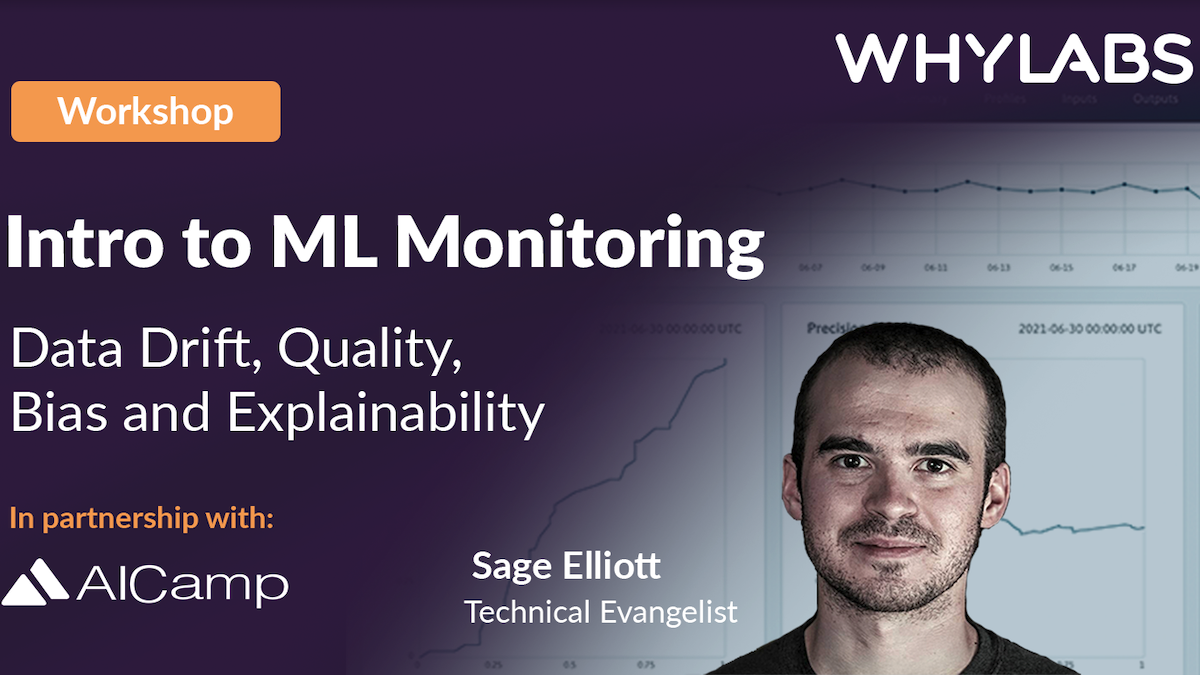
If you want to build reliable pipelines, trustworthy data, and responsible AI applications, you need to validate and monitor your data & ML models!
In this workshop we’ll cover how to ensure model reliability and performance to implement your own AI observability solution from start to finish. We will cover:
- Detecting data drift
- Measuring model drift
- Monitoring model performance
- Data quality validation
- Measuring Bias & Fairness
- Model explainability
What you’ll need:
- A modern web browser
- A Google account (for saving a Google Colab)
- Sign up free a free WhyLabs account (https://whylabs.ai/free)
Who should attend:
Anyone interested in AI Observability, Model monitoring, MLOps, and DataOps! This workshop is designed to be approachable for most skill levels. Familiarity with machine learning and Python will be useful, but not required.
By the end of this workshop, you’ll be able to implement data and AI observability into your own pipelines (Kafka, Airflow, Flyte, etc) and ML applications to catch deviations and biases in data or ML model behavior.
You will receive a certificate for completing workshop.