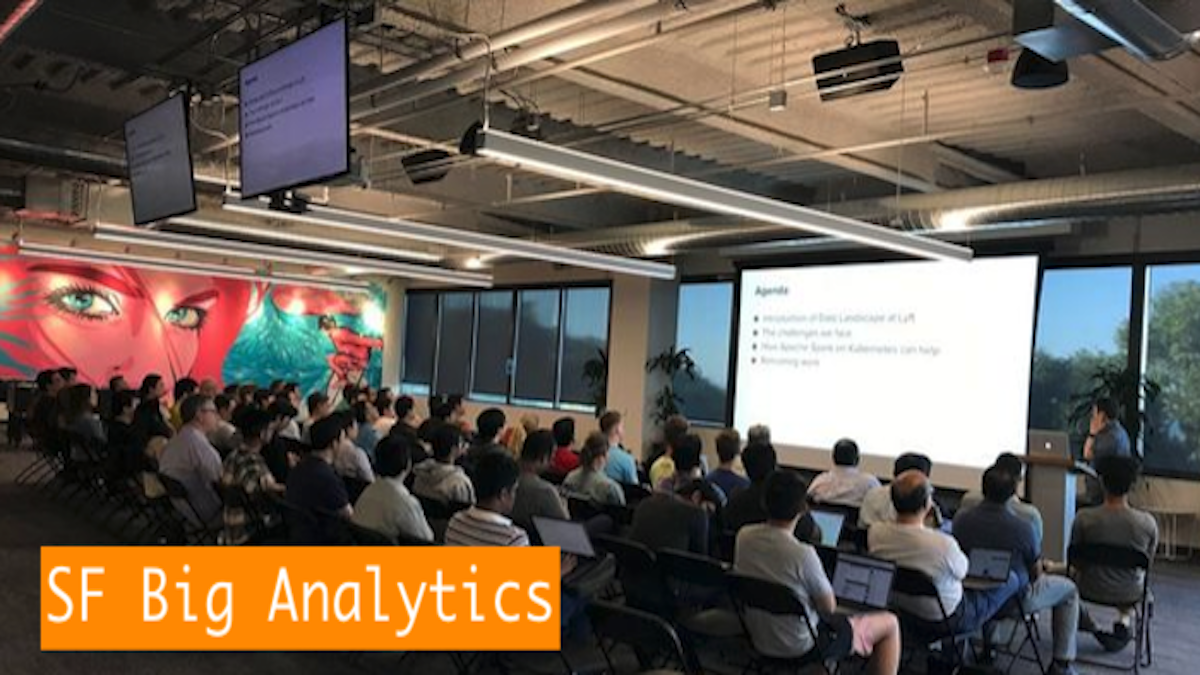
It is recognized that the role of a data engineer becomes key in the execution of data strategies. Data engineers are fully dedicated to critical tasks such as ingesting, preparing, and manipulating data needed to accomplish strategic goals. These goals are reached using various approaches such as statistical learning, deep learning, statistical modeling, etc.
But... what happened when data is not matching expectations, or even worse, when expectations are not known, or even , when downstream usage is unknown?
In this talk, Andy will demonstrate why and how frustration is created along the execution of data strategies without applying common-sense best practices. For this, he will do a live root cause analysis session using docker, SQL, Python, CSVs, and friends.
When you will feel enough of his frustration, Andy will conclude with data observability best practices to generate metadata, lineage, and metrics to avoid most of the struggles in production and share #protips to automate their implementation (e.g., in Spark and Pandas)..