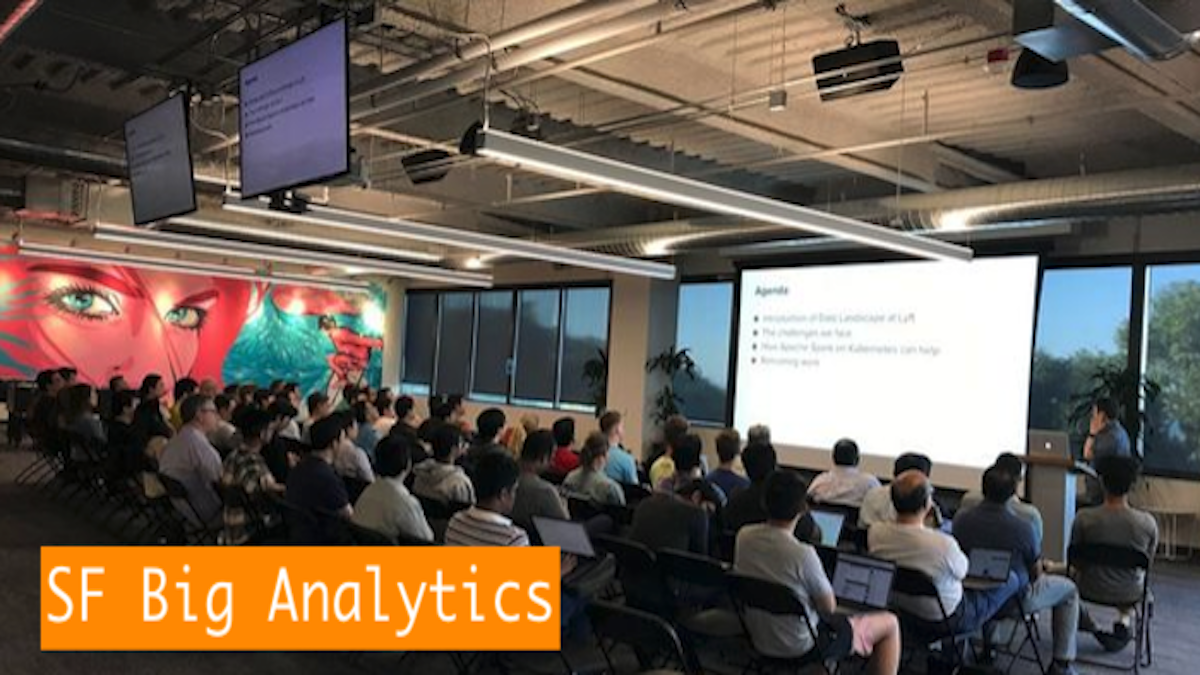
The ‘short-video’ has been proven to be one of the most successful product forms in the 4G era, witnessed by Tiktok, Instagram, Snapchat, Kuaishou, etc. AI has demonstrated its huge potentials to improve traditional businesses and create new product features in `short-video’ apps, e.g., beautifying filter, live stream, recommendation, Ads, games, risk control, etc. The common issue in the `short-video’ era faced by AI applications is the high volume of data (imagine that each user generates a behavior for every 15-20 seconds) and the huge size of the model (imagine that a recommendation model may have dozens of trillion parameters). Therefore, the acceleration of AI applications in the ‘short-video’ era greatly depend on the efficiency of the process from model training to inference and online serving.
This speaker, rely on his experiences at Kuaishou, will mainly introduce two *Pytorch* based AI engines to accelerate the training process in two major scenarios: Bagua for the “dense” scenario (e.g., CV/NLP/speech) and Persia for the sparse scenario (recommendation/Ads/search). To pursue the extreme efficiency, both engines conduct joint optimization and design of algorithms and system implementation. Bagua improves the popular open source tools such as Horovod by 30% efficiency; Persia is 7 times faster than other open sourced engines such as XDL by Alibaba, and is capable of scaling up to 100 trillion recommendation model parameters.