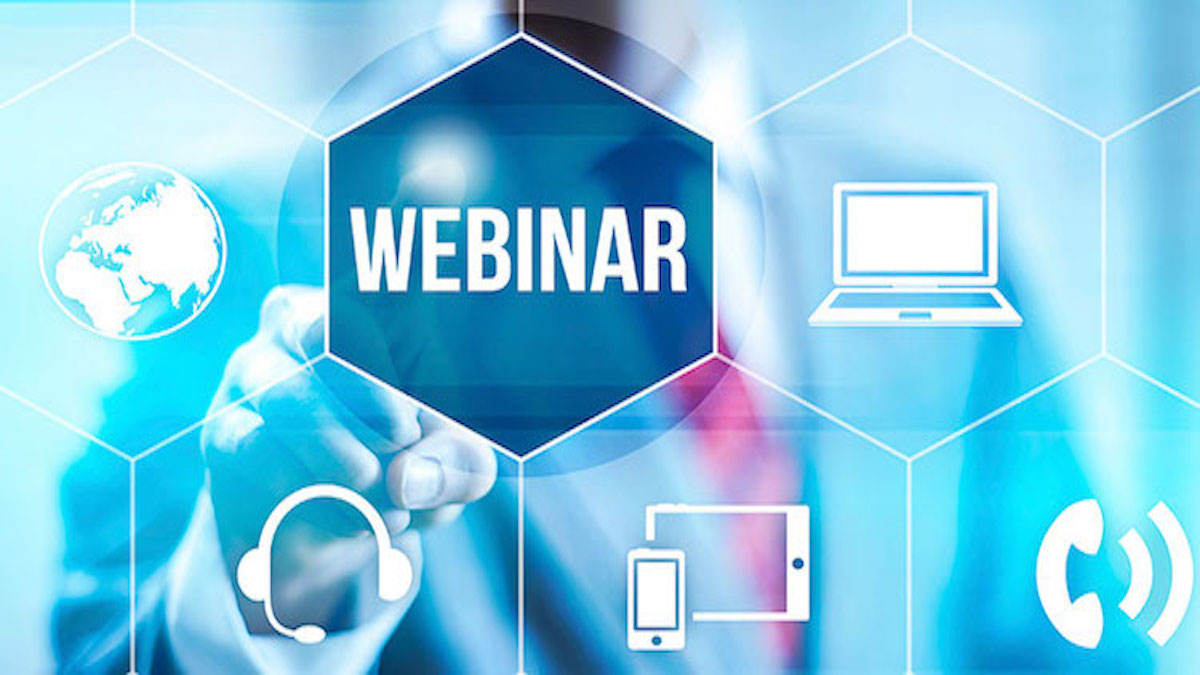
In this talk, we will walk machine learning practitioners through guidelines for efficient hyperparameter optimization based on Oríon, an open source HPO framework. We will start by presenting practical approaches for the design of the search space, then provide guidelines to select hyperparameter optimization algorithms, and finally demonstrate how to leverage the pioneering Experiment Version Control provided by Oríon for more efficiency.
Xavier Bouthillier
a PhD student advised by Pascal Vincent at Université de Montréal, Mila.
He also works as a research developper in the Innovation, Development and Technology team
at Mila, a world renowned research institute in artificial intelligence which rallies more than 500
researchers specializing in the field of deep learning. He is leading the development of the hyperparameter
optimization framework Oríon (orion.readthedocs.io).
.