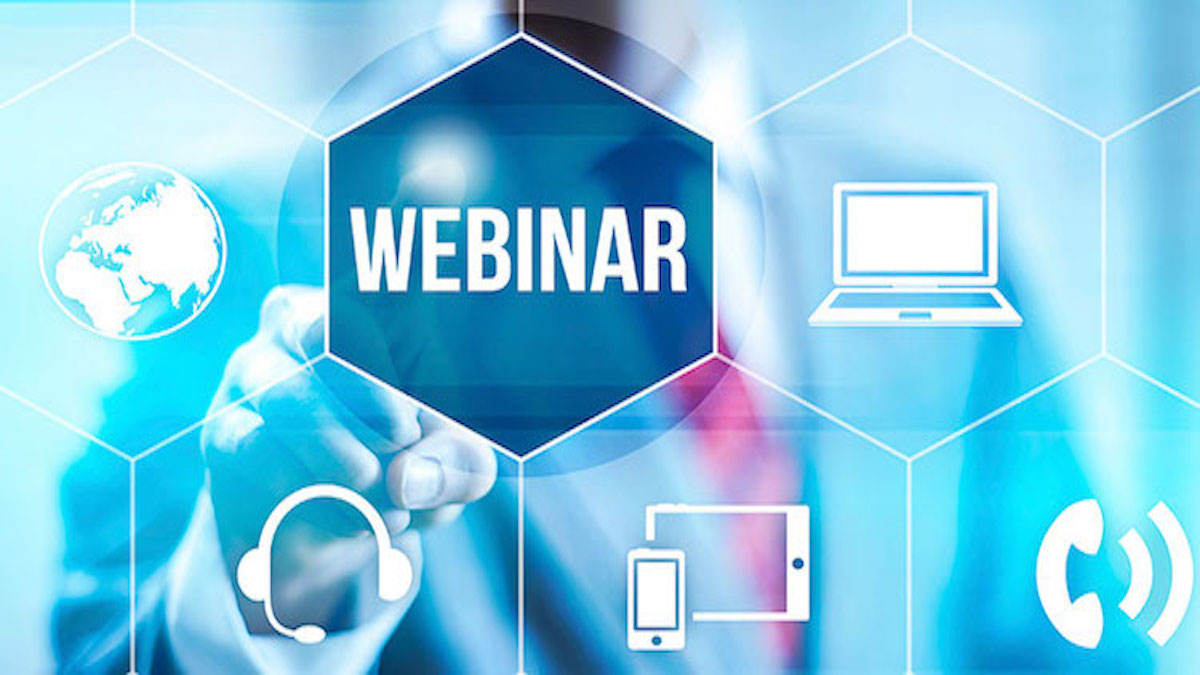
Generative Adversarial Net (GAN) is a powerful machine learning model, and becomes extremely successful recently. The generator and the discriminator in a GAN model competes each other and reaches the Nash equilibrium. GAN can generate samples automatically, therefore reduce the requirements for large amount of training data. It can also model distributions from data samples. In spite of its popularity, GAN model lacks theoretic foundation.
In this talk, we give a geometric interpretation to optimal mass transportation theory, explain the relation with the Monge-Ampere equation, and apply the theory for the GAN model, In more detail, we will discuss the following problems:
Based on this theoretic interpretation, we propose an Autoencoder-Optimal Transportation map (AE-OT) framework, which is partially transparent, and outperforms the state of the arts.